This research group is part of the Graduate School of Excellence advanced Manufacturing Engineering (GSaME).
Project description
Information and communication technology (ICT) has changed manufacturing companies in recent years and will continue to play a growing role in the future, as can be witnessed by current trends such as Industry 4.0 and digitization. The junior research group "ICT Platform for Manufacturing" at the Graduate School of Excellence advanced Manufacturing Engineering (GSaME) is responding to this trend with its central contribution: the "Stuttgart IT Architecture for Manufacturing" (SITAM). SITAM offers an integration environment for heterogeneous information systems and their data, thereby spanning different organizational units across a company. Based on this, SITAM makes it possible to analyze all structured and unstructured data in a holistic way. Furthermore, it helps to involve employees more closely into manufacturing processes via mobile devices and mobile information provisioning.
Current research activities of the junior research group primarily enhance the capabilities offered by SITAM for data preprocessing, data analysis, and machine learning. In addition to its head, the research group includes doctoral researchers of the GSaME who have a direct link to the research topic (see figure). In accordance with the dual principle of the GSaME, the group effectively combines the development of innovative scientific results with application-oriented problems. This is evident, among other things, from the fact that several of the involved doctoral projects are carried out in cooperation with an industry partner.
In its application-oriented research projects, the junior research group examines data and their potential for data analysis in various phases of a product life cycle. In product development, for example, heterogeneous simulation data can be analyzed to derive recommendations for improving a product design. In the production phase, analysis of sensor data from test benches can improve fault diagnosis and identification of quality problems for defective products. Furthermore, data from product usage can be used to predict whether and when a particular product is likely to fail. In each case, the questions arise which methods for data preparation and data analysis are to be used for specific kinds of data and how these methods must be combined in analysis processes. Here, it becomes apparent that the specific characteristics of available industrial data pose fundamental challenges for common data analysis methods. In data-driven fault diagnosis in production, as example, often very little data is available that is of sufficient quality to be used for data analysis. Likewise, the high product diversity and variability in companies increases the number and complexity of patterns and correlations contained in data.
By addressing such data characteristics, a number of fundamental research questions arise. The junior research group contributes to answering these questions by developing novel approaches tailored to the specific characteristics of industrial data. One central approach exploits domain knowledge represented by a product hierarchy to conquer the challenge of product diversity and the complexity of patterns contained in data. Moreover, the group develops methods to combine data-driven machine learning models (ML models) with physical simulation models or other knowledge-based models created by engineers. Here, the goal is to increase the accuracy and reliability of predictions in a decision support system. Another research topic is to develop an adequate metadata management to handle large heterogeneous data sets. In this context, this metadata also describes ML models trained with data and links these ML models to their application context. This metadata management enables domain experts to easily search for and explore data and ML models that are suitable for their domain-specific problems. Another approach uses the metadata to help users select and configure appropriate machine learning algorithms and other data preparation techniques.
The following projects are part of the research group "ICT Platform for Manufacturing":
- Vitali Hirsch: Data-driven Identification of Potential Quality Issues in Complex Assembly Products (completed)
- Cornelia Kiefer: Quality Assessment and Improvement in Text Analysis Pipelines (completed)
- Christian Weber: Platform to Manage Machine Learning Models in the Context of Industry 4.0 (completed)
- Alejandro Villanueva: Methods for the Design, Configuration and Selection of Machine Learning Solutions in Manufacturing (completed)
- Marco Spieß: Data-driven Prediction of Product Failures with Consideration of Dataset Shifts (running)
- Yannick Wilhelm: Hybrid Approaches to Fault Detection and Diagnosis: Combining Data-driven Models with Knowledge-based Models (running)
- Julian Ziegler: Metadata Model to Describe CAE Data and Work Activities in Virtual Product Development Projects (running)
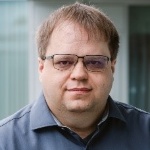
Peter Reimann
Dr. rer. nat.Researcher