This project is part of the research group ICT Platform for Manaufacturing.
Project description
High quality data and data analysis results are a precondition for future concepts such as the data-driven factory. The quality of business decisions is directly influenced by the quality of data and analysis results. Current data quality concepts and tools only consider the raw input data of data analysis pipelines. They fail to regard specifics of analysis tools as well as data characteristics for each step of analysis pipelines. To fill this research gap, this thesis presents the QUALM concept for continuous and holistic data quality measurement and improvement within data analysis pipelines.
Existing quality metrics measure the data quality of structured data, e. g., by counting null values, duplicates or invalid values. Equivalent approaches for textual data are missing. Additionally, most domain-specific text data sets are unlabeled. Thus, in addition to missing data quality metrics, also evaluation metrics are not calculable for these data sets and the derived analysis results. This leads to a high uncertainty of analysts with respect to the quality of data and analysis results. QUALM conquers this challenge with a set of concrete text data quality methods. QUALM data quality indicators quantify text characteristics and give hints with respect to the expected quality of analysis results. The QUALM indicators thereby characterize texts with respect to, e.g., the number of abbreviations, spelling mistakes, the confidence of standard analysis tools and the fit of semantic resources employed by analysis tools.
Moreover, the selection of appropriate training data is especially difficult for analysts such as domain experts with little IT and/or data science knowledge. Yet, the appropriate selection of training data has a high impact on the quality of analysis results. The corresponding QUALM indicator measures data quality by means of the similarity between input data and training data. The counterpart QUALM modifier automatically selects the best-fitting training data and thus impedes low-quality results of domain-specific analysis. Finally, QUALM offers a hybrid method for information extraction, which exploits both structured and unstructured information sources. To this end, structured data is employed as a basis for a first grouping of free text fields and for removing information from the free texts which is already present in the structured fields. Thus, the hybrid approach yields more new and relevant information.
The QUALM concept and methods are evaluated with respect to several industry-near application scenarios, such as the analysis of downtimes on a production line. In further application scenarios, sample citizen data scientists are focused, i. e., domain experts with little IT and data science knowledge, who want to build analysis pipelines from scratch.
Funding
This project was funded by the German Research Foundation (Deutsche Forschungsgemeinschaft - DFG) and the Ministry of Science, Research and Arts of the State of Baden-Württemberg as part of the Graduate School of Excellence advanced Manufacturing Engineering (GSaME).
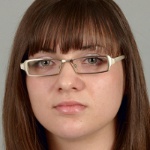
Cornelia Kiefer
Dr.-Ing.Researcher
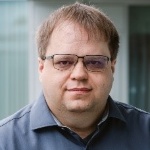
Peter Reimann
Dr. rer. nat.Researcher
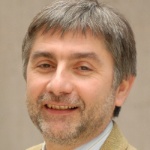
Bernhard Mitschang
Prof. Dr.-Ing. habil.Head of Institute