This project is part of the research group ICT Platform for Manaufacturing.
Project description
In the quality management of products, the increasing technical complexity and variety of products is leading to an increase in the number of quality problems. Quality problems are resolved by experienced employees, which in many cases requires several rework and repair attempts. In high-wage countries such as Germany, these additional efforts lead to significant additional costs and reduce the profitability of manufacturing companies. This dissertation presents a recommendation system that reduces the number of ineffective rework attempts that are carried out on complex assembly products, such as a combustion engine, after these products have been tested on a test bench. The recommendation system suggests product components that are the cause of a quality issue in a product and that thus should be replaced. The identification of the components is formalized as a data-driven classification problem.
Data characteristics have a significant impact on the performance of classification methods and thus on their fitness for use. Therefore, the characteristics of quality-related manufacturing data from operational practice and resulting analytical challenges are presented in this dissertation. Data from quality management, for example, are characterized by low volume and an inherent variance. These challenges may negatively affect data-driven classification algorithms. The author examines existing learning algorithms and data pre-processing techniques to determine the extent to which they can address these challenges. He proposes several novel approaches that effectively address all challenges. This mainly includes a classification system that uses domain knowledge to systematically pre-process data, leading to a higher classification accuracy.
The developed classification system is used for the first time at one of the world's largest commercial vehicle manufacturers in the End-of-Line testing area of complex assembly products. This case study shows the performance of the novel classification system. The classification accuracy of the system exceeds the accuracy of standard algorithms. The classification system assists experienced workers in identifying defective components that are the cause of a product quality issue. This way, it reduces the amount of ineffective rework attempts by about a half.
Collaboration Partner and Funding
This project was funded by the Daimler Truck AG as part of the Graduate School of Excellence advanced Manufacturing Engineering (GSaME).
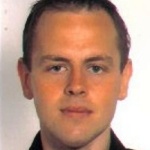
Vitali Hirsch
Dr.-Ing.Researcher
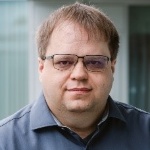
Peter Reimann
Dr. rer. nat.Researcher
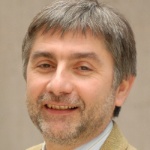
Bernhard Mitschang
Prof. Dr.-Ing. habil.Head of Institute