This project is part of the research group ICT Platform for Manaufacturing.
Project description
In the context of digitization, companies are exploring how to effectively use machine learning to implement data-driven use cases. One central domain of application is the prediction of product quality. In this context, this research project investigates how vehicle manufacturers may effectively use machine learning to predict vehicle failures. Here, early vehicle failures within the warranty period are of particular interest, given that these early failures cause high costs for the replacement of vehicle components and that they lead to customer dissatisfaction. It is important to note that different variants exits for individual components of a vehicle, such as the engine, transmission or clutch. These variants may be assembled alternatively and influence the probability of a vehicle failure. The idea is to use machine learning techniques to learn from the data from vehicles which are already in use by customers. This data analysis shall identify which combinations of assembled component variants are particularly prone to failure. The analysis results may then be applied to vehicles that are yet to be produced. This way, the component variants for these vehicles may be selected in such a way that the vehicles are more robust and do not fail during the warranty period.
This machine learning problem is characterized by high complexity due to the availability and characteristics of the data. In this context, two challenges arise that have to be addressed equally. First, vehicles fail only after a certain period of time. As a result, the real patterns for vehicles that are at risk of failure are not visible in the data until a certain amount of time has passed. As another challenge, the configuration of ordered vehicles changes over time, so that diversity in the data has to be addressed as well. These challenges fit into the scientific context of different types of dataset shifts.
In this project, existing approaches from literature to deal with dataset shifts were analyzed with respect to their suitability to address the above challenges. This study revealed that incremental and window-based approaches each address one challenge in isolation, but they never tackle both challenges. Therefore, different data pre-processing and classification techniques were tested and an approach was developed that already provides good results in predicting vehicle failures. The next step is to iteratively improve this approach with the goal of developing a holistic approach that addresses both challenges simultaneously
Collaboration Partner and Funding
This project is funded by the Daimler Truck AG as part of the Graduate School of Excellence advanced Manufacturing Engineering (GSaME).
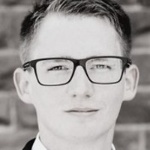
Marco Spiess
M.Sc.Researcher
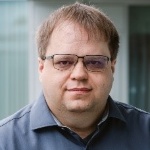
Peter Reimann
Dr. rer. nat.Researcher
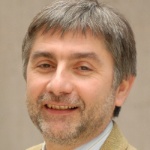
Bernhard Mitschang
Prof. Dr.-Ing. habil.Head of Institute