Post-Silicon Validation (PSV) is the last step in semiconductor manufacturing in which tests occur on the actual devices to ensure compliance to given specifications. Thereby, engineers only have limited internal observability of the device and cannot directly modify the silicon chip. Thus, in practice, a device has to be regarded as a black box. Today, PSV is typically done manually, which is a laborious and expensive task. Moreover, the problem is getting more complicated with increasing design complexity, e.g., an increasing number of transistors and other components on modern chips. Therefore, it is extremely promising to automate the PSV tuning process.
A core aim of PSV is to tune parameters of the tested chips and micro-controllers. Device-specific settings have to be found so that the chips work reliably and robustly in their later life-cycle. This tuning is difficult, as it has to cope with variations and faults in the manufacturing process. In practice, it often relies on the expertise of engineers. In the future, tuning will get even more difficult, as complexity of chips and circuits increases.
Our aim is to automatize the PSV tuning task and to cope with less assumptions as well as less or no expert knowledge by actively learning from test data. Methods that we use include Deep Neural Networks, Reinforcement Learning, Active Learning, and novel approaches to optimization.
This project is a collaboration with Advantest, one of the world-leading companies in Automated Test Equipment. Furthermore, it is part of the Graduate School “Intelligent Methods for Test and Reliability” (GS-IMTR) of the University of Stuttgart. Together with Advantest, we investigate both theoretical and practical implications in PSV.
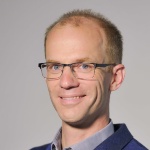
Dirk Pflüger
Prof. Dr. rer. nat.Head of Institute
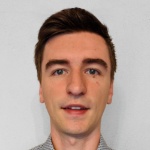
Peter Domanski
M.Sc.Researcher